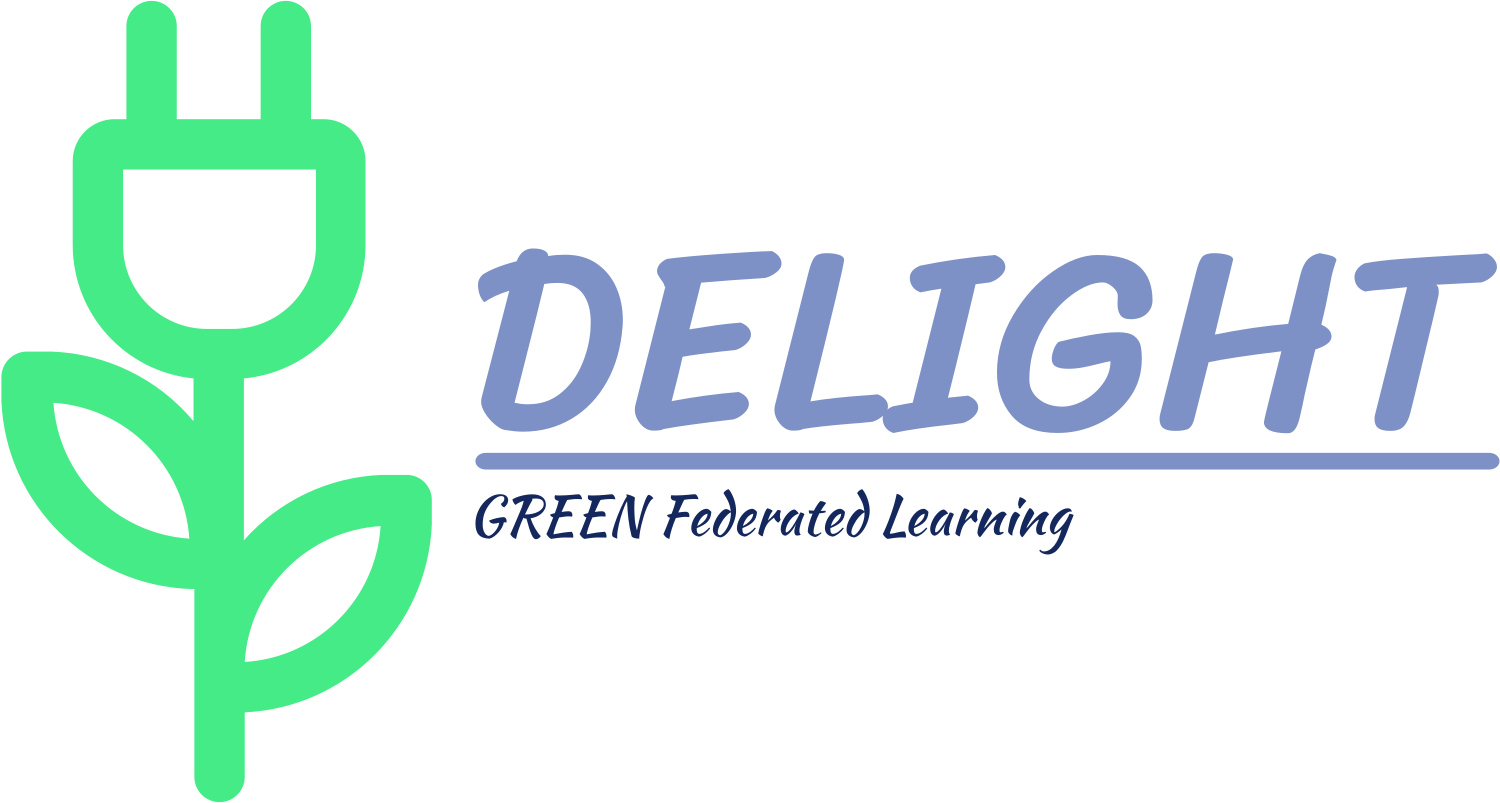
This growing demand for Federated Learning (FL) technology opens new challenges in addition to those that appear in traditional ML. These include (i) communications and energy costs between the nodes and the server; (ii) increased duration of the training period due to correlation between databases (Independent and Identically Distributed (IID) versus non-IID.); (iii) fair distribution of computation and communication between heterogeneous nodes; (iv) absence of reliable mechanisms for nodes to evaluate the benefits of joining FL; (v) FL is limited to a star topology and it is not adapted to a large-scale networks scenario. In all these challenges, energy efficiency is totally absent in all new FL architectures such as cross-node FL, cross-Silo FL, federated transfer learning, personalised FL, etc.
AI technologies today are too energy-intensive to be compatible with our sustainable development objectives. While recent work has assessed the carbon footprint of traditional learning methods, the carbon footprint of an emerging approach such as federated learning is not sufficiently studied.
The DELIGHT project aims to evaluate and reduce the energy consumption of federated learning using different levers (gradient compression, data summarization, speed-scaling, etc.). Given the heterogeneity of the data, another objective will be to study
the process of bargaining and coalition formation among nodes in order to understand to what extent a node has an interest in collaborating with others.
The techniques developed will be empirically validated on computer vision and NLP tasks using the Flower toolkit.